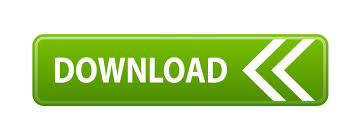
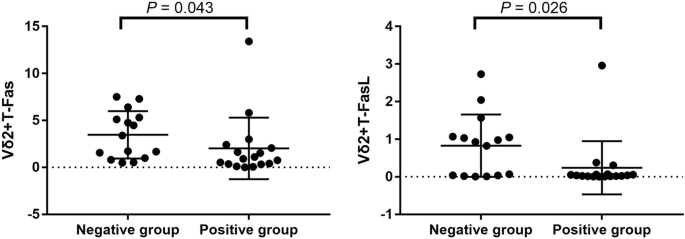
The most common approach for associating neural network components with linguistic properties is to predict such properties from activations of the neural network. In the conclusion, we summarize the main gaps and potential research directions for the field. Section 7 mentions a few other methods that do not fall neatly into one of the above themes. This is a relatively underexplored area, and we call for more work in this direction. Section 6 summarizes work on explaining model predictions, an important goal of interpretability research. We point to unique characteristics of dealing with text as a discrete input and how different studies handle them. Section 5 deals with the generation and use of adversarial examples to probe weaknesses of neural networks. In Section 4, we discuss the compilation of challenge sets, or test suites, for fine-grained evaluation, a methodology that has old roots in NLP. Section 3 discusses visualization methods, and emphasizes the difficulty in evaluating visualization work. Section 2 reviews work that targets a fundamental question: What kind of linguistic information is captured in neural networks? We also point to limitations in current methods for answering this question. It organizes the literature into several themes.
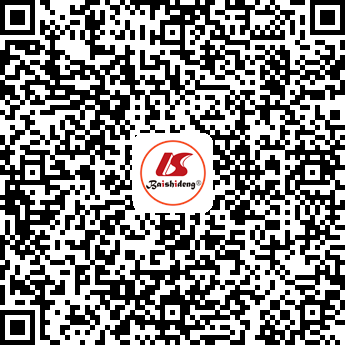
This survey aims to review and summarize this body of work, highlight current trends, and point to existing lacunae.

As the analysis of neural networks for language is becoming more and more prevalent, neural networks in various NLP tasks are being analyzed different network architectures and components are being compared, and a variety of new analysis methods are being developed.
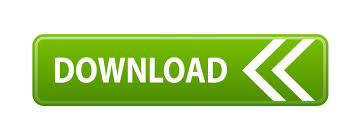